From history to fiction and real life, there are countless individuals that continually inspire and motivate us in different ways. Generative AI technologies can enable us to easily create digital portrayals of our motivators. The generated outputs can feature synthesized faces, bodies, and voices that appear and act in ways that appear natural and human. Anyone from a family member no longer with us, to a movie protagonist, scientist or a historical figure can either be created as a pre-rendered talking video or a generated face that a person can puppeteer in real-time. In this perspective, we highlight emerging use-cases of synthesized characters for increasing motivation by providing easy access to generated digital individuals that inspire us. We discuss the potential of synthetic media for positive outcomes in education and wellbeing, two critical aspects of life thrown into disarray as COVID-19 induced stay-at-home lifestyles made video conferencing the prime way to connect for work, school, fitness or socializing. We discuss how synthetic media is being used to support learning and wellbeing and demonstrate a unified pipeline that can help enable anyone to generate motivating talking heads or real-time filters from text, image, and video input. We discuss the need for inclusion of traceability for synthetic media to support machine detectability and to maintain trust. As we look towards the future, one where generative media are a part of an ever growing human-AI landscape, we foresee a need to rethink the fundamentals of what it means to be human.
TIME:
June 2020 ~ December 2020
AUTHORS:
P. Pataranutaporn,
V. Danry, P. Punpongsanon, J. Leong, P. Maes, M. Sra
KEYWORDS:
Generative AI, Learning, Well-being, Deepfakes, Speech Synthesis, Video Synthesis, Artificial Intelligence.
SKILL:
Machine Learning, GANs, Google Colab
Publication:
Pataranutaporn, P., Danry V., Punpongsanon, P., Leong, J., Maes, P., Sra, M. (2020, Under Review). Generative AI Characters for Supporting Personalized Learning and Wellbeing. In Proceedings of Nature: Machine Intelligence Journal.
June 2020 ~ December 2020
AUTHORS:
P. Pataranutaporn,
V. Danry, P. Punpongsanon, J. Leong, P. Maes, M. Sra
KEYWORDS:
Generative AI, Learning, Well-being, Deepfakes, Speech Synthesis, Video Synthesis, Artificial Intelligence.
SKILL:
Machine Learning, GANs, Google Colab
Publication:
Pataranutaporn, P., Danry V., Punpongsanon, P., Leong, J., Maes, P., Sra, M. (2020, Under Review). Generative AI Characters for Supporting Personalized Learning and Wellbeing. In Proceedings of Nature: Machine Intelligence Journal.
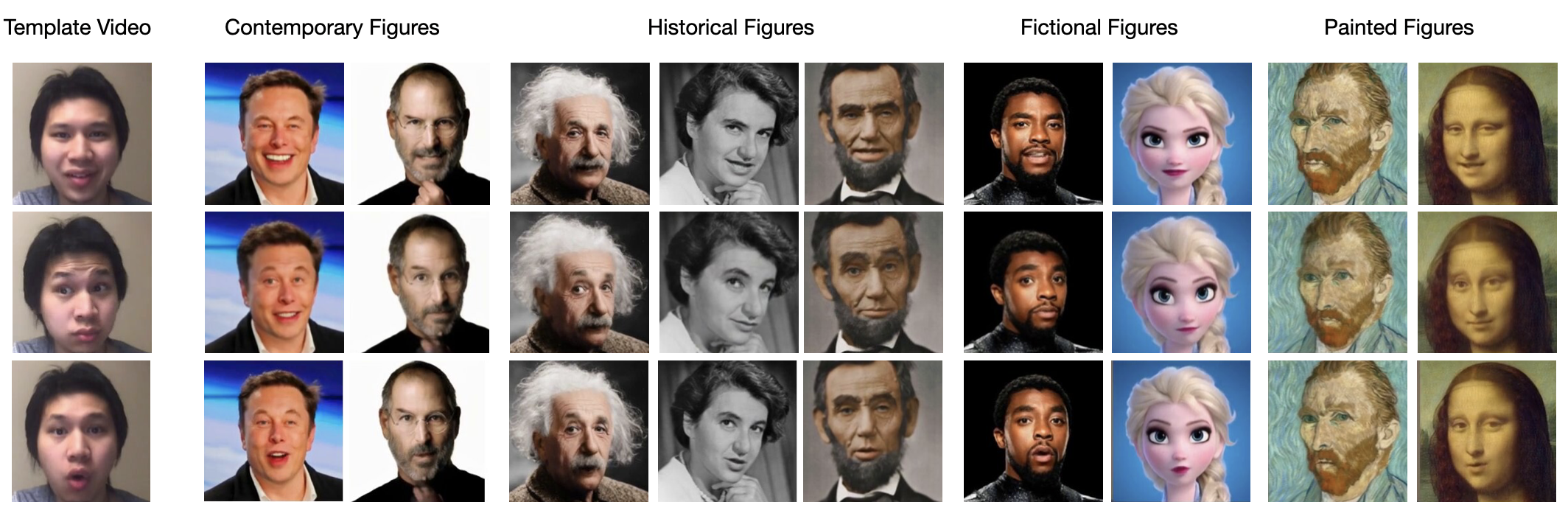
Generated characters showing a broad range of faces from contemporary humans to historical individuals, as well as fictional characters and painted art figures.
Synthetic character usage for learning can be further expanded with not only teachers but also with students playing different roles. Due to such opportunities, we see generative AI supporting a variety of venues forlifelong learning, whether that involves learning about art from the artist or learning to cook from the best chefs in the world. World renowned cellist Yo Yo Ma joined a class of geographically distributed students in Fall 2020 to discuss artistic creativity and musical composition in the time of COVID-19. A real-time AI synthesized version of Johann Sebastian Bach was generated and performed by one of the authors. Seeing his musical hero brought to life enthralled Mr. Ma and a lively discussion of Bach’s work followed. The students were deeply engaged and participated in what seemed tobe a casual conversation between longtime collaborators
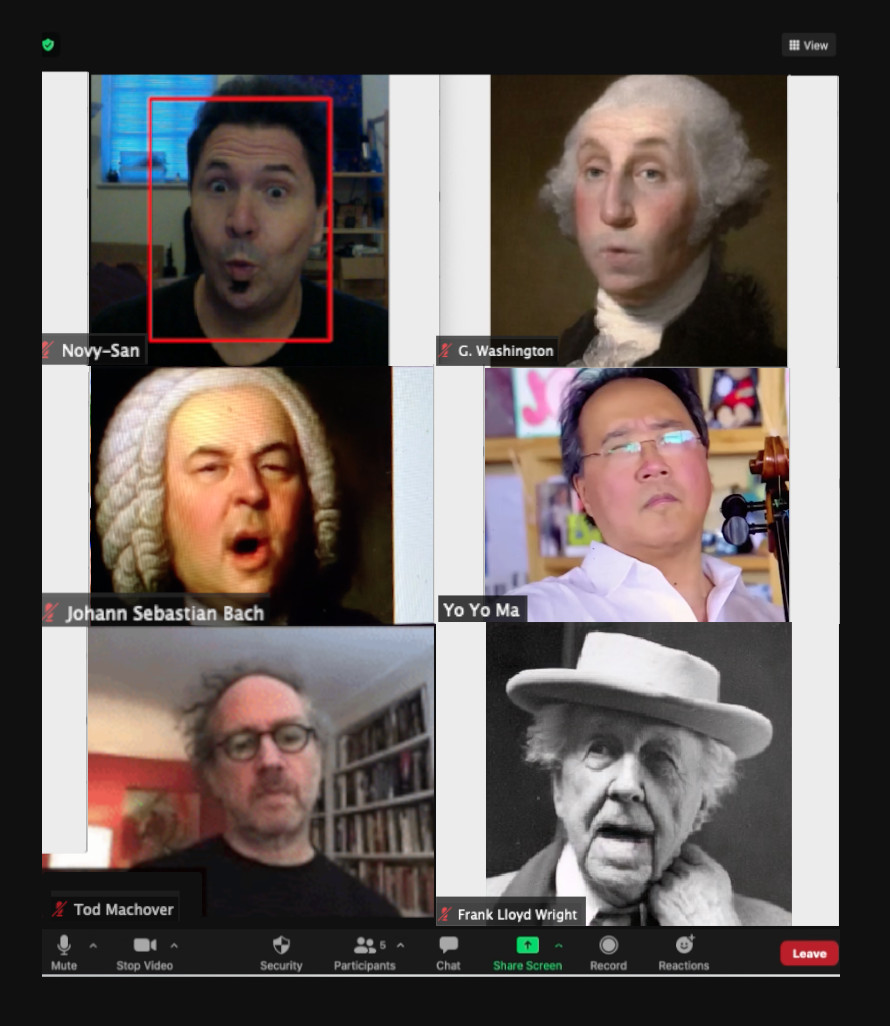
The top row shows one of the authors (left top row) as George Washington (right top row) discussing the Zoomrevival of the Tony Award winning musical ‘1776’. The middle row shows Johann Sebastian Bach talking to Yo Yo Ma in anonline class held over Zoom in Fall 2020. The bottom row shows architect Frank Lloyd Wright brought to life in another Zoomconversation.
We present a unified pipeline for enabling educators and individuals to easily generate talking characters that can be usefuladditions for educational or health applications. The pipeline allows for the easy creation of a talking head video of a human(real or fictional) or an animated human-like character with given features such as facial gestures, voice, and motion. It makesuse of four recent generative algorithms thata are combined to simplify the process of creating video output from any typeof input. The four state of the art algorithms used are: VOCA or Voice Operated Character Animation, FLAME or Faces Learned with an Articulated Model and Expressions, Speech-Driven Facial Animation, and First Order Motion Model. Our unified pipeline marks the generated results with a traceable watermark to help end users identify and learn about how the output was generated and to distinguish it from original video content. It differs from existing consumer apps in allowing any type of input media for generating video output or real-time use and provides a general purpose solution different from domainspecific apps like Pinscreen used for facial animation or ReFace used for face-swapping videos.
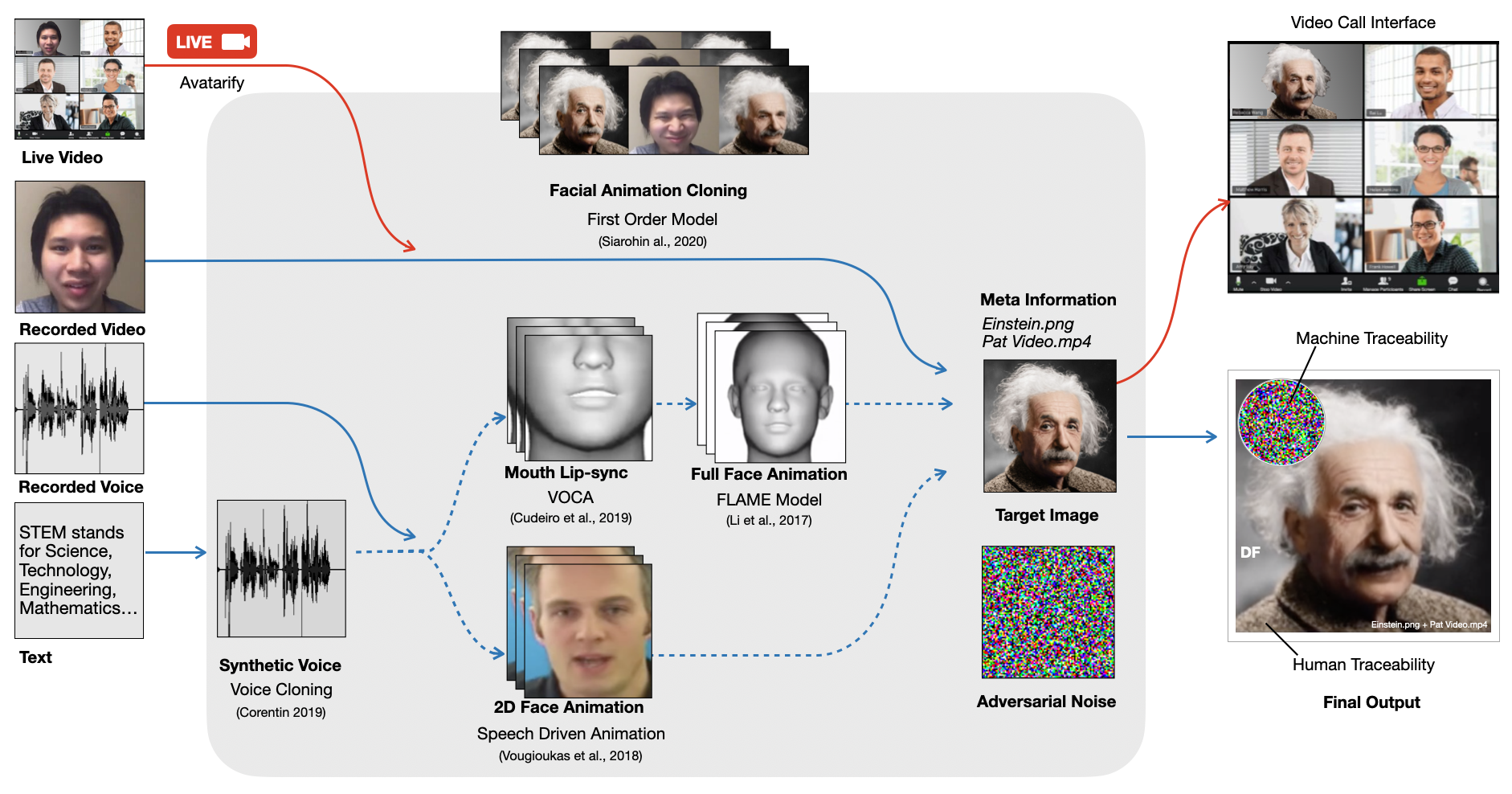
The unified pipeline that allows users to provide video, voice, or text as inputs to the system to generate both real-time and offline characters. The output is watermarked traceability (see section 4), both for algorithms and humans.